Brain diseases such as dementia impose an enormous burden to the individual and to society. As a consequence, there is an urgent need to develop effective preventive and therapeutic strategies. Early detection and accurate prediction of the progression of at-risk subjects are key in this development. Early detection is important for successful treatment and accurate prediction will play a major role in clinical trials, e.g. for selecting homogenous patient groups to reduce variability in outcome measures.
Artificial Intelligence (AI) has high potential for aiding medical decision making and creating a learning healthcare system, where current patients are diagnosed and treated based on knowledge gained from previous patients. Especially in the domain of neuroradiology, AI has proven very successful in gaining new knowledge by extracting patterns related to neurodegenerative diseases from large sets of MRI data. The research interest of the Neuroimage Analysis & Machine Learning research line is to optimally combine brain imaging, clinical data and artificial intelligence techniques to promote an accurate and early diagnosis, and eventually the right treatment, for patients with neurodegenerative disease. The group’s research focuses on development of novel biomarkers, methods for detection and prediction, and on defining the infrastructure for the development and validation of such methods. While AI is showing great experimental results and large high-quality datasets are available, methods are not yet finding their way into clinical practice. Therefore we aim to develop and collect accurate diagnostic and prediction methodology, to validate those methods on large and clinically representative datasets, to identify and overcome challenges for clinical implementation.
Three key focus areas are the development of novel neuroimaging biomarkers, the development and validation of novel AI models for accurate detection and prediction of dementia, and enabling a learning healthcare system by setting infrastructures for a.o. data sharing and federated learning.
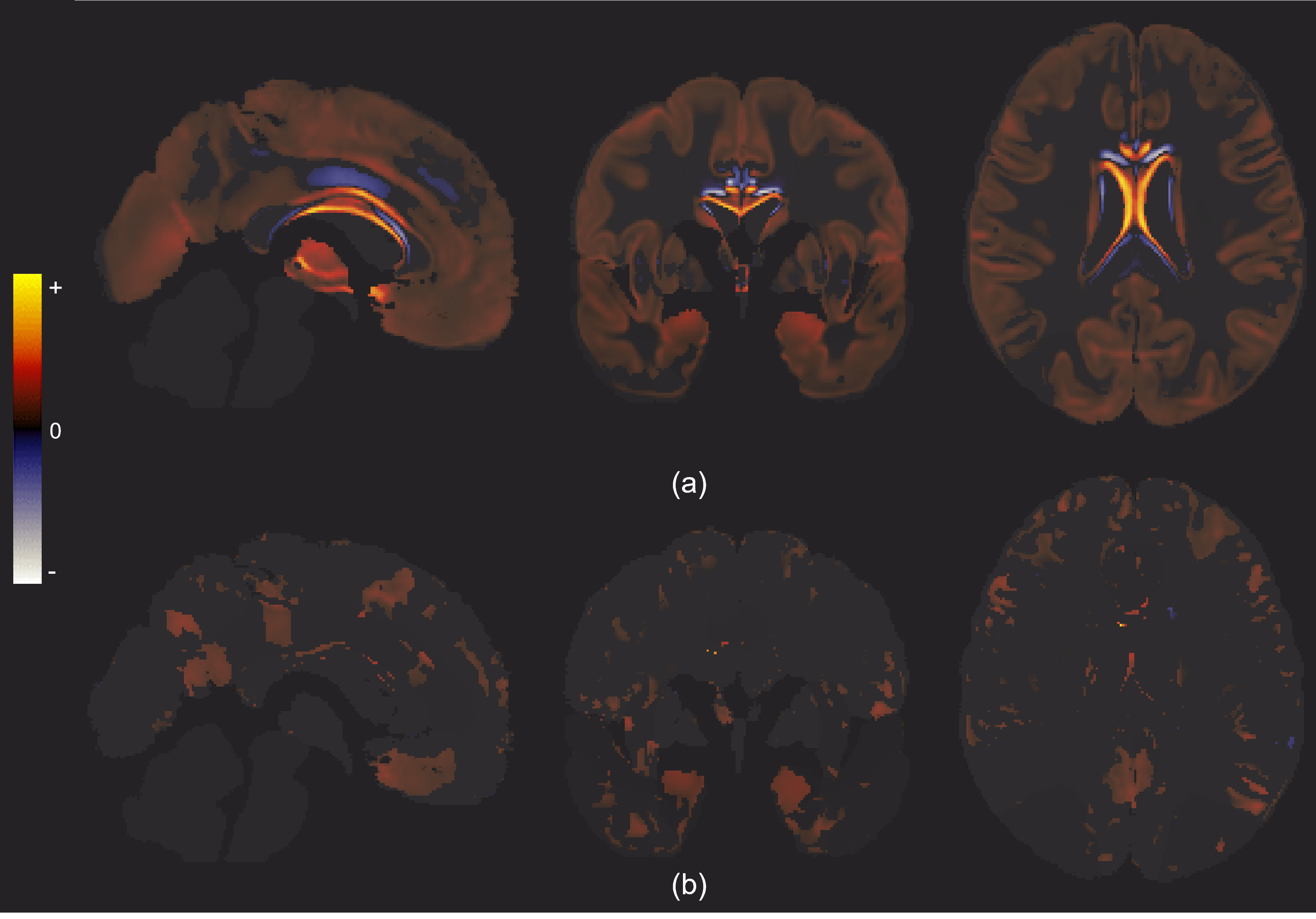
AI-enabled interpretable grey matter changes with increasing g-factor (a) without correcting for confounders, and (b) with correcting for age, sex, and education years. The results are generated using the semi-supervised model, averaged over the five folds, and masked by the significance mask.
Cardiovascular disease prediction
In addition to brain disease, we have started working on cardiovascular disease (CVD) in collaboration with Dr. Daniel Bos (Department Epidemiology and Department of Radiology & Nuclear Medicine, Erasmus MC). CVD is a leading cause of morbidity and mortality worldwide. Prevention of CVD requires accurate identification of high-risk individuals; various CVD risk prediction models have been developed to achieve primary prevention of CVD. The current standard of care employs that are commonly based on traditional regression models. However, they are limited to using a small number of well-established risk factors. They also assume that there is no interaction between the different factors and each factor has a linear relationship with the CVD outcome. To this end, machine learning (ML) including deep learning (DL) techniques may address the above limitations. They can take into account complex interactions between the factors and their non-linear effects on the outcome. Moreover, they are advantageous for exploiting imaging data such as magnetic resonance imaging (MRI) and computed tomography(CT) images. The use of ML may be advantageous to make accurate CVD risk predictions compared to traditional statistical models.
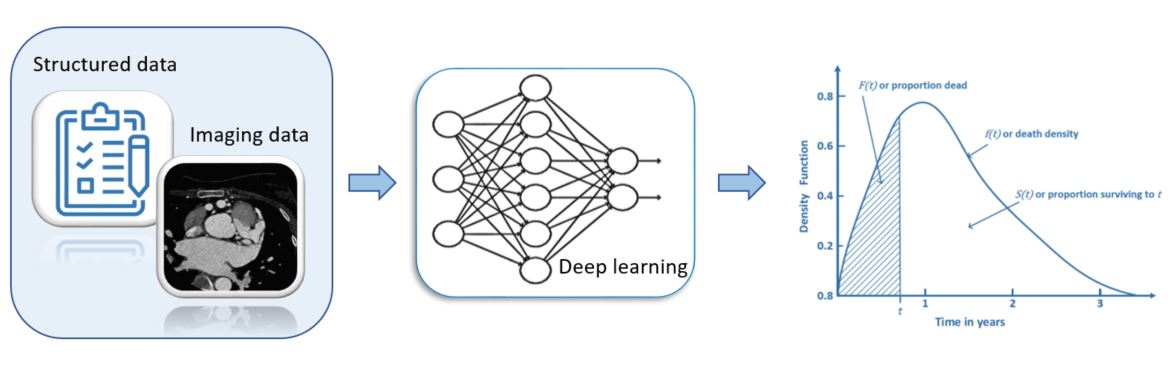
Cardiovascular disease prediction
In this research, we develop a way to leverage ML for CVD risk predictions. Considering that low-dimensional predictor data that may not result in a considerable benefit from the use of ML/DL techniques, the development will be based on additional predictors including images such as carotid MRI and multidetector CT images. Particularly, we will include different anthropometric measures such as body mass index (BMI) and a body shape index (ABSI) as well as newer risk markers discussed in a recent study, adding to the established clinical risk predictors.